63% of financial service providers are beginning to adopt Gen AI in practice, as per studies conducted by Master Of Code. The use of AI technologies in financial institutions represents more than just a fad—it signifies a substantial move toward a future that is more dependent on data, streamlined, and centred around customer needs.
As these technologies further develop, they are anticipated to transform the financial industry dramatically. Read on to delve into particular challenges financial institutions may face when integrating Gen AI into operations.
What are the applications of Gen AI adoption in financial services?
Gen AI is utilised in various ways within financial institutions, leading to significant transformations. Its applications include fraud detection, automated trading, customer service, risk management, and compliance, reshaping the financial sector by enhancing efficiency, precision, and customer-focused advancements.
-
Fraud detection and prevention
According to research by McKinsey, Gen AI adoption can help reduce fraud detection costs by 30%. The use of AI technologies has greatly improved the capacity of financial institutions to recognise and prevent fraud. With real-time monitoring and analysis, AI algorithms can examine transactions immediately, spot unusual trends and mark possibly fraudulent activities before they worsen.
Furthermore, solutions for studying behaviour with machine learning models enable these systems to understand customer actions more effectively as time goes on; this means it is simpler to identify changes in behaviour that might be linked with fraudulent conduct. This dual approach ensures a robust defence against various types of financial fraud.
-
Automated trading
Gen AI adoption has greatly influenced automated trading. AI allows for algorithmic trading, which involves executing trades quickly and in large quantities according to predetermined criteria and real-time data analysis, optimising trading strategies for improved performance. Nearly half of industry leaders are pouring resources into artificial intelligence research, according to research conducted by Master Of Code.
AI-enabled predictive analytics can forecast market trends by examining historical data and current market conditions. This ability helps traders make better-informed decisions, ultimately improving the profitability and efficiency of trading operations.
-
Customer service and experience
Enhancing customer service and experience is a key focus for financial institutions leveraging AI. Chatbots or virtual assistants can handle a large amount of customer queries while providing instant responses and help that is personalised 24/7.
AI helps create banking experiences unique to each individual by examining their data to provide tailored financial products and services. This customisation boosts customer satisfaction and loyalty, aiding institutions to create deeper and longer-lasting relations with their clients.
-
Risk management
AI is transforming risk management in financial institutions by improving the accuracy and breadth of credit scoring and portfolio management. By analysing a wider array of data points, including non-conventional sources, AI models can evaluate creditworthiness with greater precision.
Within portfolio management, Gen AI adoption is capable of continuously monitoring and optimising investment portfolios, adjusting asset allocation based on market conditions and consistently assessing risk levels. As a result, these capabilities lead to enhanced risk mitigation and more well-informed investment strategies.
-
Compliance and regulatory reporting
The integration of AI into the financial regulatory sphere, also known as RegTech, has improved the efficiency and accuracy of compliance and regulatory reporting. By automating compliance tasks, AI ensures that financial institutions comply with regulatory requirements without the need for extensive manual effort.
Additionally, machine learning can analyse vast datasets to detect regulatory breaches or anomalies, thereby substantially decreasing the risk of non-compliance. This not only aids in upholding regulatory standards but also improves the overall integrity of financial operations.
Understanding the barriers to Gen AI adoption in financial institutions
83% of companies claim that AI integration is a top priority in their business plans, according to research carried out by Exploding Topics. Businesses often prioritise adopting AI, but commonly encounter the challenge of having an inconsistent and unclear AI strategy, which hinders successful integration, accounting for almost 45% of businesses, as per the study titled, “AI adoption advances, but foundational barriers remain”, by McKinsey.
-
Regulatory challenges
When financial institutions consider implementing AI technologies, they encounter strict regulatory issues related to data privacy and compliance. The adoption of AI technologies brings forth concerns about adhering to data privacy regulations. These regulations impose rigorous guidelines for the collection, storage, and processing of personal and financial data, aiming to ensure the secure and transparent handling of customer information while safeguarding individuals' privacy rights.
The need for compliance goes beyond just data privacy and includes a wider range of regulatory structures. These structures establish requirements for the amount of available capital, liquidity ratios, and risk management procedures.
AI adoption must comply with these regulations to ensure the stability of financial systems and the protection of consumers. Regulatory authorities also stress the importance of transparency and fairness in AI decision-making processes, intending to reduce the potential for biased outcomes in areas like credit scoring and fraud detection.
-
Data complexity and quality
The very nature of financial data carries challenges because it is complex, varied, sensitive and has serious consequences if misused. Financial institutions handle large amounts of organised data such as transaction records or market information, and manage unstructured data like customer interactions and sentiments expressed on social media platforms. The problem lies in the difficulty of combining these various types of information into dependable insights which leads to uncertainties about its consistency, correctness and dependability.
In finance, maintaining the quality of data is very important. High-quality data is crucial for training AI models correctly and producing dependable predictions. Financial information is unique because it's sensitive and has regulatory impacts on the field, which requires robust data governance practices to maintain integrity and compliance with regulatory standards.
-
Risk and security concerns
The use of AI in financial institutions brings up concerns about risks to stability and security. If there's a problem with algorithmic trading, the market could become unstable because of sudden changes caused by AI strategies not working properly or reacting strangely under certain market situations. There might be bias in the models that AI uses for credit scoring, which could keep unfair lending methods going on and affect how inclusive finance is as well as consumer belief.
Cybersecurity is also a big worry in the field of AI, with AI systems becoming targets for high-level cyber attacks that want to change and steal financial data or disrupt operations. Making sure security and data protection measures are strong in these AI systems is very important for keeping finances stable and safeguarding sensitive information.
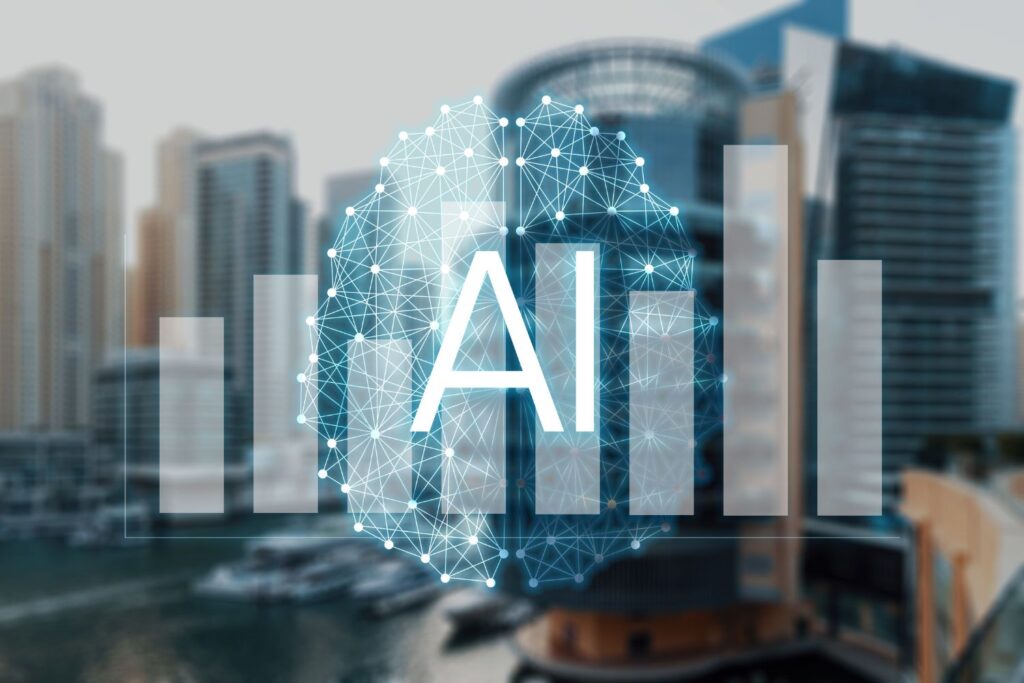
What are the strategies for overcoming barriers?
To mitigate these risks, financial institutions must establish robust risk management frameworks that align with AI technologies. This entails thorough testing and validation of AI models to identify and mitigate biases and errors before implementation. Continuous monitoring and maintenance of AI systems are essential to adapt to evolving threats and regulatory changes.
Governance, which involves clear guidelines and structures, is critical for assigning responsibilities and roles pertaining to AI oversight. This encompasses ethical standards and compliance with regulatory requirements. Furthermore, ensuring transparency in the decision-making processes of AI enhances accountability and fosters trust among involved parties. As a result, it also bolsters financial stability and security considerations.
It’s crucial for tackling any regulatory difficulties, maintaining high-quality data and handling risks in a way that leads to successful incorporation and lasting expansion within this new AI-based financial environment.
1. Ensuring AI solutions comply with regulatory requirements
According to PwC’s 2021 Global AI Survey, 64% of financial services executives identified regulatory uncertainty as a significant barrier to AI adoption. This highlights the challenges financial institutions face in integrating AI, including concerns about data privacy and compliance with existing regulatory frameworks.
Monitoring and adherence to rules: Make a special team that continuously checks regulatory changes and evaluates how they affect AI projects. Regularly update AI models and procedures to match with changing financial rules.
Cooperation with regulators: Encourage transparent communication and cooperation with regulatory groups. Participate in talks to define what regulators anticipate from AI technology, and ask for help about how to use AI in a compliant manner.
Standards of the industry and best practices: Follow the standards of your industry and best practices supported by regulatory bodies as well as industry associations. Take part in industry forums, hackathons and webinars to learn about new trends and rules from regulators.
2. Enhancing data quality and managing complexity
According to Deloitte’s report, data quality and integration challenges are significant barriers for many organisations’ Gen AI adoption, with nearly half of the respondents from the financial sector highlighting these issues as major hurdles.
Data quality assurance: Apply strict data quality assurance procedures to confirm the precision, entirety and trustworthiness of financial information. Utilise methods for cleansing data to rectify inconsistencies and errors in it.
Data governance frameworks: Set up strong data governance frameworks that outline the roles, duties, and guidelines for managing data. Make sure to follow rules about data privacy and ethics that control how the information is used.
Advanced analytics: Utilise complicated analytics methods, like machine learning and predictive modelling, to obtain useful understanding from intricate financial information, which can improve the process of making decisions and stimulate innovation in business.
3. Addressing security concerns associated with AI adoption
Security and privacy concerns are considered the two greatest barriers to broader adoption by 39% of enterprise leaders, as per a study conducted.
Measures for cybersecurity: Use strong cybersecurity methods, like encryption, access control and systems to detect intrusion. This is to safeguard AI systems and important financial information from cyber dangers.
Ethical AI practices: Establish moral guidelines for AI creation and usage. Make certain AI decision-making is clear, answerable, and unbiased to lessen biases while supporting fairness.
Integration with risk management: Utilise AI in the present risk management structures to spot, evaluate and lessen dangers linked with AI technologies. Apply comprehensive risk assessments as well as situation analysis for predicting possible risks connected to AI and creating active mitigation methods.
Financial institutions can overcome barriers to Gen AI adoption by ensuring that solutions align with regulatory requirements, improving data management practices, and effectively addressing security concerns.
They must implement strategies such as collaborating with regulators and industry bodies, adopting data governance frameworks, and integrating AI into existing risk management practices to ensure compliant, efficient, and secure deployment of AI technologies in the financial sector. These strategies not only help mitigate risks but also promote innovation and sustainable growth in the AI-driven financial landscape.
A real-life example of how financial institutions can leverage advanced technology to enhance operational efficiency and customer experience. Citibank accomplished the adoption of Gen AI into its customer service operations, significantly enhancing the customer experience through automation and personalisation.
AI-powered chatbots and virtual assistants:
- Immediate assistance: Citibank uses chatbots and virtual assistants driven by AI to assist customers with various queries and problems. These AI tools can handle basic transactions and provide a smooth and effective customer service experience.
- 24/7 support: Citibank uses AI to ensure customers receive support 24/7, leading to faster response times and improved customer satisfaction.
Personalisation and continuous improvement:
- Customer feedback and sentiment analysis: Citibank uses AI to analyse customer feedback and sentiment, enabling the bank to constantly enhance its services and meet customer needs and preferences effectively.
- Enhanced customer experience: The AI-powered solutions not only automate routine interactions but also customise the customer experience by recognising and adjusting to individual customer preferences.
The implementation of Gen AI in Citibank's customer service operations has led to:
- Increased customer satisfaction due to reduced wait times and personalised interactions.
- More efficient handling of customer queries and transactions, freeing up human agents to focus on more complex issues.
- Continuous improvement of services based on real-time feedback and sentiment analysis.
Lead your financial servicing with NayaOne
NayaOne provides advanced solutions aimed at revolutionising financial services. Our state-of-the-art technologies enable you to simplify processes, improve customer interactions, and make better decisions. By using NayaOne’s financial technology, you can confidently manage complex regulations, leveraging advanced analytics and strong security features to promote growth and effectiveness. Embrace the forefront of financial technology innovation and become an industry leader with NayaOne.