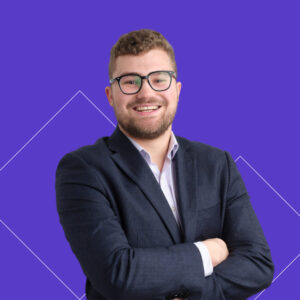
Oli Platt
Head of Client Solutions
Whether you want it or not, you can’t continue without AI in banking. From personalised banking services and fraud detection to risk management and regulatory compliance, AI is revolutionising every aspect of the banking sector. Adopting AI has become imperative to stay competitive, enhance operational efficiency, and deliver superior customer experiences.Let’s explore the transformative potential of AI in driving innovation and reshaping the banking industry.
Use Cases of AI in Banking
Artificial Intelligence (AI) has revolutionised traditional operations and initiated a new era of efficiency and innovation. Here are some ways in which banks are using AI:
Cybersecurity and Fraud Detection
AI-powered algorithms play a crucial role in enhancing cybersecurity measures within banks. These algorithms continuously analyse vast amounts of data to detect anomalies and identify potential security threats in real time. By monitoring patterns of transactions and user behaviour, AI systems can flag suspicious activities and prevent fraudulent transactions before they occur. This proactive approach not only safeguards sensitive customer information but also helps banks maintain trust and credibility in the digital realm.
Chatbots
AI-driven chatbots leverage natural language processing (NLP) and machine learning algorithms to interact with customers in a human-like manner. They can address queries, provide account information, and offer personalised recommendations. By deploying chatbots across various channels, including websites and mobile apps, banks can provide round-the-clock assistance. They can streamline communication and enhance overall customer satisfaction.
Loan and Credit Decisions
AI algorithms are increasingly being used to assess creditworthiness and make informed lending decisions. They can analyse vast datasets encompassing financial history, credit scores, and transaction patterns. Because of this, AI models can predict the likelihood of loan defaults and assess risk factors more accurately than traditional methods. This enables banks to offer personalised loan products, streamline approval processes, and mitigate credit-related risks effectively.
Data Collection and Analysis
anks generate and process enormous volumes of data on a daily basis, ranging from customer transactions to market trends. AI technologies enable banks to streamline data collection, analysis, and interpretation processes. As a result, they can extract valuable insights to drive informed decision-making. By leveraging advanced analytics and machine learning algorithms, banks can identify patterns, detect anomalies, and derive actionable intelligence from complex datasets. Thus, they can optimise operations and enhance performance.
Customer Experience Enhancement
AI plays a pivotal role in enhancing the overall customer experience by automating routine tasks, personalising interactions, and streamlining service delivery. From automated KYC (Know Your Customer) processes to personalised product recommendations, AI-driven solutions enable banks to cater to the unique needs and preferences of individual customers, thereby fostering stronger relationships and loyalty.
Risk Management
AI-powered risk management systems enable banks to assess and mitigate various forms of risk, including credit risk, market risk, and operational risk. By analysing historical data, market trends, and external factors, AI models can predict potential risks and vulnerabilities. This enables banks to implement proactive measures and safeguard against financial losses.
Challenges in Adopting AI in Banking
While the adoption of AI holds immense promise for the banking industry, it is not without its share of challenges. Overcoming these hurdles is crucial for banks to realise the full potential of AI and leverage its benefits. Here are some of the key challenges in adopting AI in banking and financial services:
Data Security
One of the primary concerns in adopting AI technology in banking is ensuring the security and privacy of customer data. Banks deal with vast amounts of sensitive information, including personal and financial data, which makes them lucrative targets for cyberattacks. Ensuring robust data security measures, including encryption, access controls, and threat detection systems, is essential to safeguard against data breaches and unauthorised access.
Lack of Quality Data
When using generative AI in banking, the effectiveness of AI models depends heavily on the quality and relevance of the underlying data. However, many banks struggle with fragmented data sources, inconsistencies, and inaccuracies, which can hinder the performance of AI algorithms. Addressing data quality issues and ensuring access to clean, reliable data is critical for training accurate and reliable AI models. Alternatively, synthetic data can help banks both improve the quality of data as well as boost security.
Lack of Understanding
AI algorithms, particularly deep learning models, often seem like black boxes. This makes it challenging to interpret and understand their decisions and actions. Lack of explainability can lead to distrust among customers, regulators, and internal stakeholders, especially in critical areas such as credit decisions and regulatory compliance. Banks must prioritise transparency and accountability in AI-driven processes, ensuring that decisions are explainable and traceable.
Regulatory Compliance
The banking industry is subject to stringent regulatory requirements and compliance standards, which can pose significant challenges for AI adoption. Banks must ensure that AI systems comply with regulations such as GDPR, PSD2, and Basel III, while also adhering to industry-specific guidelines and best practices. Achieving regulatory compliance requires careful planning, risk assessment, and ongoing monitoring of AI systems. In addition, the need to comply with emerging AI regulations, such as the EU AI Act, put an additional strain on banks.
Talent Acquisition and Training
Building and maintaining AI capabilities requires a skilled workforce with expertise in data science, machine learning, and AI. However, there is a growing shortage of AI talent in the banking industry. Therefore, it is challenging for banks to recruit and retain qualified professionals. Investing in talent development programs, partnerships with relevant institutions, and collaboration with technology vendors can help address this challenge.
Legacy Systems and Infrastructure
Many banks grapple with outdated legacy systems and infrastructure that are ill-equipped to support AI initiatives. Integrating AI technologies into existing systems requires significant investment in modernisation and digital transformation efforts. Banks must overcome technical debt, streamline data architecture, and embrace cloud-based solutions to create a robust foundation for AI implementation.
Resistance to Change
Adopting AI involves organisational change and cultural transformation, which can meet resistance from employees, stakeholders, and traditional decision-making processes. Overcoming resistance to change requires effective change management strategies, leadership commitment, and clear communication of the advantages of AI in banking.Encouraging collaboration, fostering a culture of innovation, and providing training and support are essential for driving successful AI initiatives.
Embracing an AI-First Approach
Embracing an AI-first approach has become imperative for staying competitive and meeting the evolving needs of customers. An AI-first approach involves prioritising the integration of artificial intelligence technologies across all aspects of banking operations, from customer service to risk management. Here are key steps for banks to embrace an AI-first approach:
Developing an AI Strategy
The journey towards an AI-first approach begins with developing a comprehensive AI strategy that aligns with the bank’s goals, vision, and values. This strategy should outline the objectives for AI adoption, identify key use cases, and define the roadmap for implementation. Collaborating with cross-functional teams, including IT, data science, and business units, is essential for ensuring alignment and buy-in across the organisation.
Investing in AI Talent and Capabilities
Building a strong foundation for AI adoption requires investing in talent and capabilities to develop, deploy, and maintain AI-driven solutions. Banks need to recruit data scientists, machine learning engineers, and AI specialists with expertise in banking and finance. Additionally, investing in training programs, upskilling initiatives, and partnerships with academic institutions can help build internal AI capabilities.
Fostering a Culture of Innovation
Embracing an AI-first approach requires fostering a culture of innovation and experimentation within the organisation. Banks should encourage employees to explore new ideas, challenge conventional thinking, and embrace emerging technologies. Creating dedicated innovation labs, organising hackathons, and incentivising creativity can help drive a culture of continuous learning and improvement.
Collaborating with Fintech Partners
Collaborating with fintech partners and technology vendors can accelerate the adoption of AI and bring innovative solutions to market faster. Banks can leverage the expertise and specialised capabilities of fintech startups to complement their internal efforts and address specific business challenges. Building strategic partnerships with fintech companies can also provide access to cutting-edge technologies, market insights, and new revenue opportunities.
Ensuring Regulatory Compliance and Ethical AI Practices
As banks embrace AI-driven technologies, ensuring regulatory compliance and ethical AI practices becomes paramount. Banks must adhere to regulatory guidelines, data privacy laws, and industry standards governing the use of AI in finance and banking.Implementing robust governance frameworks, conducting regular audits, and establishing ethical guidelines for AI development and deployment are essential for building trust with customers, regulators, and other stakeholders.
Measuring and Monitoring AI Performance
Effective measurement and monitoring of AI performance are critical for evaluating the impact of AI initiatives and driving continuous improvement. Banks should establish key performance indicators (KPIs) aligned with business objectives, such as customer satisfaction, operational efficiency, and risk management. Regularly monitoring AI performance against these KPIs enables banks to identify areas for optimisation, address challenges, and make data-driven decisions.
Scaling AI Adoption Across the Organisation
Scaling AI adoption across the organisation requires a systematic approach and strong leadership commitment. Banks should prioritise investments in scalable AI infrastructure, data platforms, and governance mechanisms. Additionally, fostering a culture of collaboration, knowledge sharing, and cross-functional teamwork is essential for driving successful AI adoption and integration across all business units and functions.
What to Expect in the Future of AI in Banking?
The future of AI in banking holds immense promise. Continued advancements promise to reshape the industry and redefine how banks operate, engage with customers, and manage risks. As technology evolves and customer expectations continue to rise, several key trends will shape the future landscape of AI in finance and banking:
Personalised Banking Experiences
AI-powered solutions will enable banks to deliver highly personalised and tailored experiences to customers. By leveraging data analytics, machine learning algorithms, and predictive modeling, banks can anticipate customer needs, offer personalised product recommendations, and deliver proactive financial advice.
Conversational Banking
Chatbots, virtual assistants, and natural language processing (NLP) technologies will play a central role in enabling conversational banking experiences. Customers will interact with AI-powered bots through voice commands, messaging platforms, and virtual interfaces. This will enable seamless communication, faster resolution of queries, and enhanced customer engagement.
Enhanced Fraud Detection and Cybersecurity
AI-based fraud detection and cybersecurity solutions will become increasingly sophisticated. They will leverage advanced analytics, anomaly detection, and real-time monitoring to identify and mitigate emerging threats. Banks will deploy AI-driven algorithms to detect fraudulent transactions, authenticate user identities, and safeguard sensitive data against cyberattacks, thereby enhancing security and trust.
Robotic Process Automation (RPA)
RPA technologies will continue to automate manual and repetitive tasks across banking operations. This will drive efficiency, reduce costs, and improve productivity. Banks will deploy AI-powered bots to streamline back-office processes, automate account opening procedures, and accelerate loan approvals, enabling employees to focus on higher-value tasks and strategic initiatives.
Hyper-Personalised Financial Advice
AI-driven wealth management platforms will provide hyper-personalised financial advice and investment recommendations. By analysing vast amounts of data, including market trends, economic indicators, and customer preferences, AI algorithms will help optimise investment portfolios, mitigate risks, and maximise returns for clients.
Regulatory Compliance and Risk Management
AI technologies will enhance regulatory compliance and risk management practices within the banking industry. Banks will leverage AI-driven analytics, natural language processing, and predictive modeling to ensure adherence to regulatory requirements, detect compliance violations, and mitigate financial risks in real time.
Ethical AI and Responsible Innovation
As AI adoption accelerates, banks will increasingly focus on ethical AI principles, responsible innovation, and algorithmic transparency. Organisations will prioritise fairness, accountability, and transparency in AI-driven decision-making processes, ensuring that algorithms are free from bias, discrimination, and unintended consequences.
Fostering Innovation and Collaboration with NayaOne's AI Sandbox
An AI Sandbox can drive experimentation and collaboration. It provides a controlled environment where banks can test and validate AI-powered solutions, algorithms, and models without disrupting their core operations or risking regulatory compliance.
An AI Sandbox offers various benefits:
- Foster Innovation: An AI Sandbox enables banks to explore new ideas and technologies in a risk-free setting.
- Increasing Safety: By providing access to anonymised data and simulation tools, banks can experiment with different AI algorithms and techniques, gaining valuable insights into their performance and potential impact on business outcomes.
- Accelerate Innovation Cycles: This iterative approach allows banks to rapidly prototype and refine AI solutions, reducing time-to-market for new products and services.
- Promote Collaboration and Knowledge Sharing: By inviting external stakeholders such as fintech startups, research institutions, and regulatory bodies to participate in sandbox initiatives, banks can tap into a diverse pool of expertise and perspectives.
- Ensure Compliance: An AI Sandbox promotes responsible AI adoption and ensures compliance with relevant laws and regulations, helping banks assess potential risks and vulnerabilities, address ethical considerations, and demonstrate robust governance frameworks to regulators and stakeholders.
As banks continue to embrace AI as a strategic differentiator, the role of AI sandboxes will become increasingly critical in shaping the future of banking innovation.
Ready to Unlock the Power of AI in Banking?
At NayaOne, we’re committed to empowering financial institutions with the tools and resources they need to thrive in the AI-first world. Our AI Sandbox platform provides a secure and collaborative environment for testing and refining AI solutions, enabling you to unlock new opportunities, enhance customer experiences, and drive sustainable growth.
Ready to take your banking innovation to the next level? Contact us to learn more about our AI Sandbox solutions and start your journey towards AI-powered success. Together, we can shape the future of banking and create value for customers, stakeholders, and society as a whole.